Bringing a digital fish to life
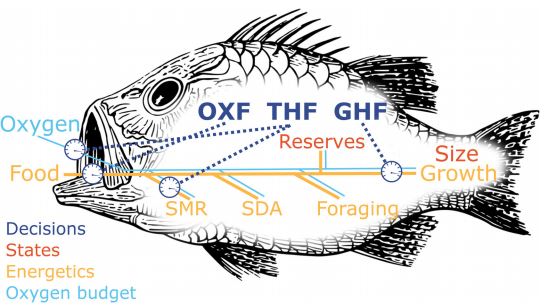
Towards the digital fish: WHY?
In many fields of research, there are high-quality digital models of physical systems, to the extent that they have been called a digital twin. If the digital model of a system represents a true digital copy of the physical system, it is possible to save both time and costs in performing experiments on the digital twin before on the physical system. A digital twin of a fish would in addition reduce the need for experiments with live animals, which is a central goal for ethical experimentation (Flecknell P 2002. Replacement, reduction and refinement. ALTEX 19:73-78).
A digital twin of a fish is probably not achievable. First, a fish is not a human construction, so there is no blueprint to copy. Second, even a single cell is immensely complex, with its state being partly controlled by its past and partly by maybe tens of thousands of genes. Thirdly, a cell or a fish is only partly understood. But while the digital twin of a fish may be impossible to build, we may still aspire to come close to building it, both for scientific curiosity and for ethical experimentation. At least we may aim to have rich models of the main parts of it.
There has been a long tradition in science of taking the opposite approach, named after the medieval philosopher-theologian William of Ockham (“Occam’s razor”): to make theories only as complex as needed to explain a phenomenon. This has led to many models of biological systems with just a handful or less of model parameters. However, if one tries to compare the output of a simple model with that of a complex biological system, or even tries to tweak the simple model to produce the same system behaviour as the biological system, then the few parameters in the explicit model must also take the burden to represent all the implicit factors: those that exit in the natural world but not in the model. Yet, it is not possible to include all factors, as we neither know their existence nor their dynamics. Our philosophy has been to move from simplicity towards more complexity, also because simple models can only generate simple dynamics. To discover unexpected properties and processes which may emerge in a model requires more complex model descriptions.
Towards the digital fish: development, evolution and environment
To model an organism, we need two or even three approaches (Giske & al. 1998). We need models of the components in the organism, such as sensing, physiology etc (Budaev & al. 2024). We also need models of how evolution has formed its priorities, such as behaviour, life history and growth pattern. And we need to model the environment around the organism. As movement is a core defining ability of animals, space use is an excellent narrative for integrating these three aspects of being a fish (Giske & al. 1998).
Premises for a digital twin of the Atlantic salmon in its world: agency, robustness, subjectivity, and prediction
Aquaculture, Fish and Fisheries. 4: e153. [ doi:10.1002/aff2.153 ] [ open access ] [ pdf ]
Modelling spatial dynamics of fish
Reviews in Fish Biology and Fisheries. 8: 57-91. [ pdf ]
Towards the digital fish: our building blocks
Jump to section:- Agent-Based Modelling and evolution by machine learning
- Modular and degenerant behavioural architectures simplify adaptive evolution
- Cognition and the architecture for decision-making
- Fish welfare
- Hormonal control of the phenotype
- Sensing, feeding, growth and mortality risk
- Learning and sociality
- Fish personalities and behaviour
- Evolution of adaptive behaviour by the ING method
- Models of other marine life than fish
Agent-based modelling and evolution by machine learning
Most of our simulation models for decision-making utilize machine learning (ML) to arrive at adaptive behaviour. Here, ML is embedded within an agent-based model (ABM) of a population that evolves and adapts over a number of discrete generations. The central component in the ML is a genetic algorithm that sends genes of those individuals who have lived in a way that has made them grow up and become parents off to the next generation. Thus, for each generation, the combinations of alleles in the genomes of individuals that become parents, will advance into the next generation. Due to sexual reproduction, the genomes of the offspring will be a mix of its two parents. In addition, mutations can happen. At first, we used the genetic algorithm to evolve the strengths in an artificial neural network (The ING method, see below). Later, we have represented emotions and cognition more biologically, rather than via a neural network (Giske & al. 2013, Budaev & al 2018). We have also coded for genes that control life history decisions directly (Fiksen 2000).We have done much research on how to find adaptive solutions to complex evolutionary trade-offs of individuals in agent-based models, where we first developed the ING method (see below). Our motivation for developing this method, and our later methods development, is that we explore solutions to "problems" that are too hard to solve by analytical methods. Therefore, we have evolved the solutions gradually over many generations. Later, we have tried to mimic the decision mechanisms in animals better than by a neural network. Now, we code aspects of the architecture that feeds into cognition and behaviour as "genes" (see "Cognition and the architecture for decision-making" below), and use a genetic algorithm to evolve adaptive solutions (Giske & al 2003, 2013, 2014, Eliassen & al 2016, Budaev & al 2018, 2019).
We have also participated in an international effort led by Volker Grimm and Steve Railsback for developing standardised protocols for documentation of agent-based models, to make it easier for other researchers to find the information needed to repeat the research (Grimm & al 2006, 2010, 2020, Stillman & al 2015). This has also improved the quality of the modelling.
The ODD protocol for describing agent-based and other simulation models: A second update to improve clarity, replication, and structural realism
Journal of Artificial Societies and Social Simulation. 23(2): 7. [ doi:10.18564/jasss.4259 ] [ open access ] [ pdf ]
Decision-making from the animal perspective: Bridging ecology and subjective cognition
Frontiers in Ecology and Evolution. 7: 164. [ doi:10.3389/fevo.2019.00164 ] [ open access ] [ pdf ]
AHA: A general cognitive architecture for Darwinian agents
Biologically Inspired Cognitive Architectures. 25: 51-57. [ doi:10.1016/j.bica.2018.07.009 ] [ open access ] [ pdf ]
From sensing to emergent adaptations: Modelling the proximate architecture for decision-making
Ecological Modelling. 326: 90-100. [ doi:10.1016/j.ecolmodel.2015.09.001 ] [ open access ] [ pdf ]
Making predictions in a changing world: the benefits of individual-based ecology
BioScience. 65: 140-150. [ doi:10.1093/biosci/biu192 ] [ open access ] [ pdf ]
The emotion system promotes diversity and evolvability
Proceedings of the Royal Society B-Biological Sciences. 281: 20141096. [ doi:10.1098/rspb.2014.1096 ] [ open access ] [ pdf ] [ fortran code for model at datadryad.org ]
Effects of the emotion system on adaptive behavior
American Naturalist. 182: 689-703. [ doi:10.1086/673533 ] [ open access ] [ pdf ] [ popularized version ]
The ODD protocol: A review and first update
Ecological Modelling. 221: 2760-2768. [ doi:10.1016/j.ecolmodel.2010.08.019 ] [ pdf ]
A standard protocol for describing individual-based and agent-based models
Ecological Modelling. 198: 115-156. [ doi:10.1016/j.ecolmodel.2006.04.023 ] [ pdf ]
Explicit trade-off rules in proximate adaptive agents
Evolutionary Ecology Research. 5: 835-865. [ pdf ]
The adaptive timing of diapause - a search for evolutionarily robust strategies in Calanus finmarchicus
ICES Journal of Marine Science. 57: 1825-1833. [ pdf ]
Ecology in Mare Pentium: An individual-based spatio-temporal model for fish with adapted behaviour
Fisheries Research. 37: 163-178. [ pdf ]
Modelling spatial dynamics of fish
Reviews in Fish Biology and Fisheries. 8: 57-91. [ pdf ]
Modular and degenerant behavioural architectures simplify adaptive evolution

One of the most used modules in our models is the neuronal response function, which is a 2-gene conversion of an input strength (e.g. an environmental variable or bodily signal) to the experience of this input strength in the nervous system (Giske & al 2013, Andersen & al 2016). Machine learning by the genetic algorithm over many generations leads to adaptive allele values of these genes, as illustrates by the three shapes in the figure at right.
Modularity and degeneracy increase the robustness of the organism and the evolvability of the gene pool (Giske & al 2014).
Premises for a digital twin of the Atlantic salmon in its world: agency, robustness, subjectivity, and prediction
Aquaculture, Fish and Fisheries. 4: e153. [ doi:10.1002/aff2.153 ] [ open access ] [ pdf ]
Decision-making from the animal perspective: Bridging ecology and subjective cognition
Frontiers in Ecology and Evolution. 7: 164. [ doi:10.3389/fevo.2019.00164 ] [ open access ] [ pdf ]
AHA: A general cognitive architecture for Darwinian agents
Biologically Inspired Cognitive Architectures. 25: 51-57. [ doi:10.1016/j.bica.2018.07.009 ] [ open access ] [ pdf ]
The proximate architecture for decision-making in fish
Fish and Fisheries. 17: 680-695. [ doi:10.1111/faf.12139 ] [ open access ] [ pdf ]
The emotion system promotes diversity and evolvability
Proceedings of the Royal Society B-Biological Sciences. 281: 20141096. [ doi:10.1098/rspb.2014.1096 ] [ open access ] [ pdf ] [ fortran code for model at datadryad.org ]
Effects of the emotion system on adaptive behavior
American Naturalist. 182: 689-703. [ doi:10.1086/673533 ] [ open access ] [ pdf ] [ popularized version ]
Cognition and the architecture for decision-making

The brain as a prediction machine. By its Subjective Internal Model (SIM, Budaev & al 2019) the fish keeps and updates an image of itself and its surroundings. This image forms the basis for the prediction machine's ability to deliver an expectation to what new sensory information will bring, and also to predict outcomes of its behavioural choices. New sensory information can lead to competition among neurobiological states. Here, the winner determines the next Global Organismic State (GOS, Giske & al 2013) where attention is restricted to the current dominant brain state. As part of the decision-making, the prediction machine will re-use connections between sensing and emotion to simulate the emotional outcome of a behavioural option.
Experimenters normally use simple artificial environments and experimental systems focused on a single problem or context that are controllable and lack ambiguity. The natural environment is very different: it is usually complex, heterogeneous, continuously changing, and stochastic. What is the “context” may not be obvious for the organism. The fish is bombarded with numerous, conflicting, and often novel stimuli. A considerable fraction of incoming sensory information is irrelevant, and distracting, the relevant sensory input is often partial, inaccurate, and ambiguous. Thus, the fundamental problem of adaptive behavior and decision-making in a naturally complex environment is what are the best stimuli to respond to and what are the best contexts to choose for achieving a specific goal such as allostasis, survival, or reproduction (Budaev & al 2019).
We find the two-step survival circuit concept of Joseph LeDoux very useful. In the first part, survival circuits compete to determine the animal’s Global Organismic State. This is thus a “context” competition to determine what is the current reality: which model of the world shall the animal use now. Thereafter attention will focus on the winning context to determine an appropriate behaviour.
Lately we have focussed on the second half of this process, after a fish has decided its priorities (after it has determined which is the currently best model of reality) until it decides its behaviour. We investigate the prediction machine concept from neuroscience: that the fish will re-use its neuronal winding from sensing to emotion to simulate the likely feeling it would obtain by executing a behavioural option (Budaev & al 2019, and also Budaev & al 2018).
We first found that modelling a very simple desicion architecture can be sufficient to divide a population into genetically determined personality types (Giske & al 2013). Some may be quite distinct while others show gradual transitions. Further, the degeneracy and modularity of the architecture lead to rich genetic variation in the population that will simplify adaptation to new ecological conditions, if or when this happens (Giske & al 2014). Hence, this architecture facilitates evolvability, and central elements in the architecture are very old (Andersen & al 2016). These results are robust with respect to changes in the architecture (Eliassen & al 2016).
For more details, links to source codes etc. see The AHA Model web page.
Premises for a digital twin of the Atlantic salmon in its world: agency, robustness, subjectivity, and prediction
Aquaculture, Fish and Fisheries. 4: e153. [ doi:10.1002/aff2.153 ] [ open access ] [ pdf ]
Decision-making from the animal perspective: Bridging ecology and subjective cognition
Frontiers in Ecology and Evolution. 7: 164. [ doi:10.3389/fevo.2019.00164 ] [ open access ] [ pdf ]
AHA: A general cognitive architecture for Darwinian agents
Biologically Inspired Cognitive Architectures. 25: 51-57. [ doi:10.1016/j.bica.2018.07.009 ] [ open access ] [ pdf ]
From sensing to emergent adaptations: Modelling the proximate architecture for decision-making
Ecological Modelling. 326: 90-100. [ doi:10.1016/j.ecolmodel.2015.09.001 ] [ open access ] [ pdf ]
The proximate architecture for decision-making in fish
Fish and Fisheries. 17: 680-695. [ doi:10.1111/faf.12139 ] [ open access ] [ pdf ]
The emotion system promotes diversity and evolvability
Proceedings of the Royal Society B-Biological Sciences. 281: 20141096. [ doi:10.1098/rspb.2014.1096 ] [ open access ] [ pdf ] [ fortran code for model at datadryad.org ]
Effects of the emotion system on adaptive behavior
American Naturalist. 182: 689-703. [ doi:10.1086/673533 ] [ open access ] [ pdf ] [ popularized version ]
Explicit trade-off rules in proximate adaptive agents
Evolutionary Ecology Research. 5: 835-865. [ pdf ]
Fish welfare
The prediction machine concept and re-use of neuronal wiring (called re-entrance) creates a link between animal behaviour and animal welfare, as the normal decision-making process in a animal tries to predict its near-future emotional wellbeing (Budaev & al 2020), which in some situations can lead to stress in the animal. In salmon aquaculture in Norway, stress is a major factor in mortality in the sea phase. Stress is again connected to fish wellbeing, almost as two sides of a coin.Computational animal welfare: towards cognitive architecture models of animal sentience, emotion and wellbeing
Royal Society Open Science. 7: 201886. [ doi:10.1098/rsos.201886 ] [ open access ] [ pdf ]
Hormonal control of the phenotype
The graphical model of hormonal control of the phenotype in a growing juvenile fish, from Weidner & al (2020).
While our work in cognition has focussed on bottom-up mechanisms, we here have asked how a top-down evolutionary optimization perspective can explain the hormonal control of the organism. Thus, while most theories of animal behaviour are derived from top-down evolutionary perspectives, our models of cognition search for the bottom-up control of behaviour. And while most studies of the hormone system focus on the bottom-up effects or hormones on physiology, behaviour and life histories, we here ask for the top-down evolutionary control. Sometime in the future we hope to be able to join the sensory, cognitive and hormonal influences on the phenotype.
Our model of hormonal control has concentrated on the growth period in a juvenile fish. Since the hormone system shall answer to evolutionary fitness maximization (of course: this just means it is evolved by natural selection), the hormone system shall both consider growth and mortality risk. The basic description of the model is given in Weidner & al (2020). In Jensen & al (2021) we find that also the hormonal system can be described in terms of predictive ability, in that it tries to achieve an allostatic control that considers likely future changes that the organism can prepare for.
Adaptive host responses to infection can resemble parasitic manipulation
Ecology and Evolution. 13: e10318. [ doi:10.1002/ece3.10318 ] [ open access ] [ pdf ]
Hormonal adjustments to future expectations impact growth and survival in juvenile fish
Oikos. 130: 41-51. [ doi:10.1111/oik.07483 ] [ pdf ] [ Code on Github ]
Hormones as adaptive control systems in juvenile fish
Biology Open. 9: bio046144. [ doi:10.1242/bio.046144 ] [ open access ] [ pdf ] [ Interview with Jacqueline ]
Sensing, feeding, growth and mortality risk
We have modelled fish vision as function of light intensity in the depth (Aksnes & Giske 1993, Aksnes & Utne 1997) and of water quality (Giske & al 1994, Fiksen & al 2002). Then we have used these models to calculate the predation risk for zooplankton or other prey types in the vertical, as well as for the fish. This model of visual range has been used in very many of the other papers on decision-making and animal behaviour. In Giske & al (1998) we also describe models for many other sensory modalities, such as hearing, olfaction, and the lateral line. For study of diet choice, we have combined models of prey encounter with models of the digestion processes (Giske & al 1995, Salvanes & al 1995, Fall & Fiksen 2020). We have also used this appraoch to model growth and bioenergetics.
No room for dessert: a mechanistic model of prey selection in gut-limited predatory fish
Fish and Fisheries. 21: 63-79. [ doi:10.1111/faf.12415 ] [ open access ] [ pdf ]
Modelling and interpreting fish bioenergetics: a role for behaviour, life history traits and survival trade-offs
Journal of Fish Biology. 88: 389-402. [ doi:10.1111/jfb.12834 ] [ open access ] [ pdf ]
Predator-prey encounter and capture rates in turbulent environments
Limnology and Oceanography: Fluids and Environments. 4: 85-105. [ doi:10.1215/21573689-2768717 ] [ pdf ]
Fishing-induced evolution of growth: concepts, mechanisms, and the empirical evidence
Marine Ecology. 33: 1-25. [ doi:10.1111/j.1439-0485.2011.00460.x ] [ open access ] [ pdf ]
Evolution of growth in Gulf of St Lawrence cod?
Proceedings of the Royal Society B-Biological Sciences. 275: 1111-1112. [ doi:10.1098/rspb.2007.1429 ] [ open access ] [ pdf ]
The influence of turbidity on growth and survival of fish larvae: a numerical analysis
Hydrobiologia. 484: 49-59. [ pdf ]
Modelling spatial dynamics of fish
Reviews in Fish Biology and Fisheries. 8: 57-91. [ pdf ]
A surface-dependent gastric evacuation model for fish
Journal of Fish Biology. 47: 679-695. [ pdf ]
Why pelagic planktivores should be unselective feeders
Journal of Theoretical Biology. 173: 41-50. [ pdf ]
Visual predators, environmental variables and zooplankton mortality risk
Vie et Milieu. 44: 1-9. [ pdf ]
A theoretical model of aquatic visual feeding
Ecological Modelling. 67: 233-250. [ pdf ]
Learning and sociality
We have studied what conditions favour evolution of learning, and under which circumstances the learning strategy is better or worse than the non-learning alternative. Our key assumption is that individual learning through exploration incurs a time-cost relative to the innate or genetically fixed strategy. Some situations allow coexistence of learners and non-learners in the same population, while life expectancy may be an important determinant for the adaptive value of learning.
Quantifying the adaptive value of learning in foraging behaviour
American Naturalist. 174: 478-489. [ doi:10.1086/605370 ] [ pdf ]
Exploration or exploitation: life expectancy changes the value of learning in foraging strategies
Oikos. 116: 513-523. [ doi:10.1111/j.2006.0030-1299.15462.x ] [ pdf ]
Co-existence of learners and stayers maintains the advantage of social foraging
Evolutionary Ecology Research. 8: 1311-1324. [ pdf ]
Avoidance learning and “personality” in the guppy (Poecilia reticulata)
Journal of Comparative Psychology. 112: 92-94. [ pdf ]
Fish personalities and behaviour
Evolution of genetic variation within a population that can be described as animal personalities emerges in our models with behavioural architecture (e.g. Giske & al 2013, 2014). Sergey Budaev was a poineer in studies of animal personalities long before moving to Bergen.
A mechanistic theory of personality-dependent movement behaviour based on dynamic energy budgets
Ecology Letters. 22: 213-232. [ doi:10.1111/ele.13187 ] [ pdf ]
Individual differences in behavior and mechanisms of ecological differentiation on the example of fish
Biology Bulletin Reviews. 5: 462-479. [ doi:10.1134/S2079086415050023 ] [ pdf ]
The emotion system promotes diversity and evolvability
Proceedings of the Royal Society B-Biological Sciences. 281: 20141096. [ doi:10.1098/rspb.2014.1096 ] [ open access ] [ pdf ] [ fortran code for model at datadryad.org ]
Effects of the emotion system on adaptive behavior
American Naturalist. 182: 689-703. [ doi:10.1086/673533 ] [ open access ] [ pdf ] [ popularized version ]
Personality traits and behaviour
Pages 135-165 in Fish cognition and behavior, edited by Brown C, Laland K, Krause J. Oxford, UK: Blackwell Publishing. [ pdf ]
Shyness and behavioural asymmetries in larval zebrafish (Brachydanio rerio) developed in light and dark
Behaviour. 146: 1037-1052. [ doi:10.1163/156853909X404448 ] [ pdf ]
Habituation of predator inspection and boldness in the guppy (Poecilia reticulata)
Journal of Ichthyology. 43: 243-246. [ pdf ]
Individuality in fish behavior: Ecology and comparative psychology
Journal of Ichthyology. 42: S189-S195. [ pdf ]
Individual differences in parental care and behaviour profile in the convict cichlid: A correlation study
Animal Behaviour. 58: 195-202. [ doi:10.1006/anbe.1999.1124 ] [ pdf ]
Consistency of individual differences in behaviour of the lion-headed cichlid, Steatocranus casuarius
Behavioural Processes. 48: 49-56. [ pdf ]
Sex differences in the Big Five personality factors: Testing an evolutionary hypothesis
Personality and Individual Differences. 26: 801-814. [ pdf ]
Avoidance learning and “personality” in the guppy (Poecilia reticulata)
Journal of Comparative Psychology. 112: 92-94. [ pdf ]
How many dimensions are needed to describe temperament in animals: A factor reanalysis of two data sets
International Journal of Comparative Psychology. 11: 17-29. [ pdf ]
Difference in shoaling behaviour between ocellated (Symphodus ocellatus) and long-striped (S. tinca) wrasses and its relation to other behavioural patterns
Marine and Freshwater Behaviour and Physiology. 31: 115-121. [ pdf ]
The statistical analysis of behavioural latency measures
ISCP Newsletter. 14: 1-4. [ pdf ]
Alternative styles in the European wrasse, Symphodus ocellatus: Boldness-related schooling tendency
Environmental Biology of Fishes. 49: 71-78. [ pdf ]
“Personality” in the guppy (Poecilia reticulata): A correlational study of exploratory behavior and social tendency
Journal of Comparative Psychology. 111: 399-411. [ pdf ]
Evolution of adaptive behaviour by the ING method

ING consists of an Individual-Based Model where the decisions in each individual is controlled by its Artificial Neural Network which again is inherited from the parents and evolved by a Genetic Algorithm. Its ability to find the optimal solution has been studied by comparing with dynamic programming (Huse & al. 1999). The tool has been used to model capelin distribution in the Barents Sea (Huse & Giske 1998) and vertical migration in mesopelagic fish (Strand & al. 2002).
However, while ING is able to evolve adaptive solutions to very complex situations, the method does not consider the ability of the organisms to find these solutions. This is the main reason we continued thinking, and arrived at studying the cognitive architecture for decision-making.
Artificial evolution of life history and behavior
American Naturalist. 159: 624-644. [ pdf ]
Implementing behaviour in individual-based models using neural networks and genetic algorithms
Evolutionary Ecology. 13: 469-483. [ pdf ]
Ecology in Mare Pentium: An individual-based spatio-temporal model for fish with adapted behaviour
Fisheries Research. 37: 163-178. [ pdf ]
Modelling spatial dynamics of fish
Reviews in Fish Biology and Fisheries. 8: 57-91. [ pdf ]
Models of other marine life than fish
We have also modelled other marine animals, plants and microbes, some which are prey or competitors to fish, or have important roles in the marine ecosystems. Here are a few of them:
Body size adaptions under climate change: zooplankton community more important than temperature or food abundance in model of a zooplanktivorous fish
Marine Ecology Progress Series. 636: 1-18. [ doi:10.3354/meps13241 ] [ open access ] [ pdf ] [ Feature Article ] [ Online supplement ]
Centennial decline in North Sea water clarity causes strong delay in phytoplankton bloom timing
Global Change Biology. 25: 3946-3953. [ doi:10.1111/gcb.14810 ] [ open access ] [ pdf ]
Coupling survey data with drift model results suggests that local spawning is important for Calanus finmarchicus production in the Barents Sea
Journal of Marine Systems. 165: 69-76. [ doi:10.1016/j.jmarsys.2016.09.010 ] [ pdf ]
Dynamics of phytoplankton blooms in turbulent vortex cells
Journal of The Royal Society Interface. 14. [ doi:10.1098/rsif.2017.0453 ] [ pdf ] [ Corrigendum ]
Editorial: Modeling the plankton - Enhancing the integration of biological knowledge and mechanistic understanding
Frontiers in Marine Science. 4. [ doi:10.3389/fmars.2017.00358 ] [ pdf ]
A theoretical analysis of how strain-specific viruses can control microbial species diversity
Proceedings of the National Academy of Sciences. 111: 7813-7818. [ doi:10.1073/pnas.1400909111 ]
Optimal defense strategies in an idealized microbial food web under trade-off between competition and defense
PLoS ONE. 9: e0101415. [ doi:10.1371/journal.pone.0101415 ] [ open access ] [ pdf ]
Successful strategies in size structured mixotrophic food webs
Aquatic Ecology. 47: 329-347. [ doi:10.1007/s10452-013-9447-y ] [ pdf ]
Trait-based models of nutrient uptake in microbes extend the Michaelis-Menten framework
Reviews in Limnology and Oceanography. 58: 193-202. [ doi:10.4319/lo.2013.58.1.0193 ] [ pdf ]
Relationship between fish and jellyfish as a function of eutrophication and water clarity
Marine Ecology Progress Series. 471: 73-85. [ doi:10.3354/meps10036 ] [ open access ] [ pdf ]
Inherent and apparent traits in microbial nutrient uptake
Marine Ecology Progress Series. 440: 41-51. [ doi:10.3354/meps09355 ] [ open access ] [ pdf ]
Simulation of optically conditioned retention and mass occurrences of Periphylla periphylla
Journal of Plankton Research. 32: 773-783. [ doi:10.1093/plankt/fbq015 ] [ pdf ]
Early is better: seasonal egg fitness and timing of reproduction in a zooplankton life-history model
Oikos. 116: 1331-1342. [ doi:10.1111/j.2007.0030-1299.15893.x ] [ open access ] [ pdf ]
Predation efficiency in visual and tactile zooplanktivores
Limnology and Oceanography. 49: 69-75. [ pdf ]
The significance of optical properties in competition among visual and tactile planktivores: A theoretical study
Ecological Modelling. 98: 123-136. [ pdf ]